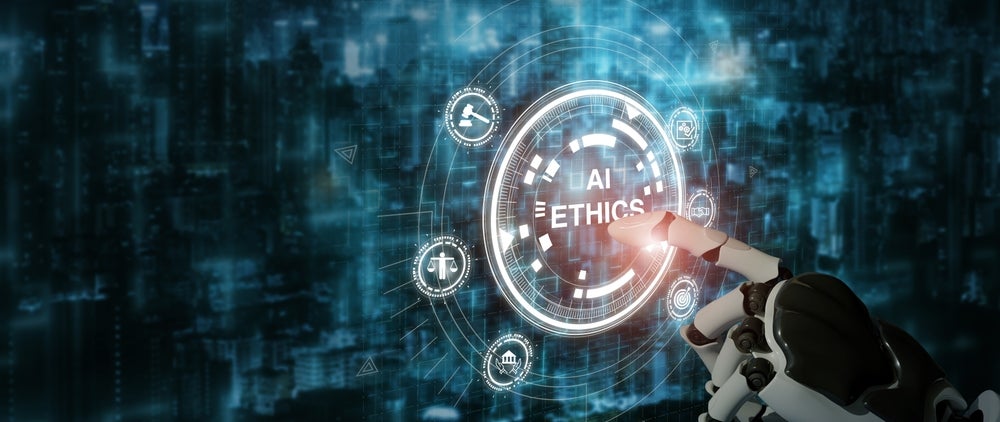
December 2023 saw the European Union reach a political agreement on the world’s first comprehensive law on artificial intelligence (AI) – the EU AI Act. This regulation places specific requirements on providers and operators of high-risk AI systems, such as testing, documentation, transparency and reporting. Financial organisations must also prepare to use tools to ensure compliance with the new regulations.
While AI offers significant benefits to the financial sector, such as personalised customer experiences or optimised risk management, it also poses ethical risks. This becomes particularly clear when AI initiatives are extended to new business areas and new standards are set for the development, use and monitoring of AI models.
We don’t know what we don’t know
Sufficient responsible AI model standards do not yet exist in business areas where AI has not been used previously. There is, therefore, a greater likelihood of poor decisions or ethical breaches. However, regardless of this situation, financial organisations have a responsibility to understand the ethical implications of their AI initiatives and take proactive measures to avoid negative impacts and a recent FICO study provides some useful insights. Conducted among 100 leading figures from the banking and financial sector, the study asked: How can we ensure that AI is used ethically, transparently and safely for the benefit of customers?
The establishment of an ethics council was cited as a solution, with 81% of respondents in the financial sector stating that they had already taken this step. The industry is clearly committed to the ethical use of this technology. However, having a committee and ensuring ethics are met are two different things.
Interpretable models on the way to trustworthy AI
In order to even begin to address ethical questions in the field of AI, it is essential that AI technologies do not remain a ‘black box’. A cornerstone of AI ethics is the interpretability of decisions made by AI systems or machine learning algorithms. Without knowledge of the underlying parameters and decision-making processes, it is impossible to assess the ethical dimension of these decisions. This creates an area of tension over which should be prioritised: the predictive efficiency or the traceability of the decision-making process? And it raises the question: How can businesses utilise the efficiency of AI systems while still ensuring they make demonstrably ethical decisions through interpretable architectures?
To engender trust in AI systems, it is necessary to understand how the underlying algorithms work. The more transparent the AI process, the greater the acceptance and trust of users. However, many companies find it difficult to understand the decision-making process of AI models, particularly in machine learning, due to the choice of the algorithms used and their inability to sufficiently explain AI decisions.
How well do you really know your competitors?
Access the most comprehensive Company Profiles on the market, powered by GlobalData. Save hours of research. Gain competitive edge.
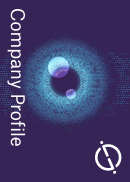
Thank you!
Your download email will arrive shortly
Not ready to buy yet? Download a free sample
We are confident about the unique quality of our Company Profiles. However, we want you to make the most beneficial decision for your business, so we offer a free sample that you can download by submitting the below form
By GlobalDataFinancial organisations should therefore replace legacy systems that have insufficient explainability and rely on interpretable AI models instead. These models make it possible to understand the relationships between the data and the resulting decisions which, in turn, enables companies to explain to their customers how the AI models work. They can also recognise and avoid bias and ensure that the decisions made by the AI systems are in line with ethical and legal frameworks.
With interpretable machine learning algorithms, a financial institution can decide for itself what can be learnt and used in the AI model. This is in contrast to “black box” machine learning, where explanations are only inferred, and often in the wrong way.
Focus on interpretability is important
With the increasing integration of AI technologies, it is crucial that financial organisations prioritise responsible and comprehensible solutions. With these in an organisation’s portfolio, the business itself and its customers can reap tangible benefits.
Transparency is also essential when it comes to information that is required for higher-risk activity such as for the automated verification and processing of loans, the detection and prevention of fraud and the optimisation of trading on stock markets. What is especially important is that this transparency is continuous. It must be monitored correctly and accurately to ensure ethically sound results.
Mitigating against model drift
As organisations have machine learning models making inferences, recognising patterns and then making predictions, it is essential that the model continues to be responsible and ethical in the light of changing data. It is not sufficient to just build a responsible AI model and let it run independently and indefinitely — it should be continually monitored to ensure its outcomes remain responsible and ethical in production.
This means that as data environments change, not only can the validity of predictions change over time, but so can the ethical use of the model. If an organisation is going to have models, it must govern and monitor them, and must know how to monitor appropriately – this should be established as a contractual requirement when the model is first built. More than a third of companies in our survey said the governance processes they have in place to monitor and re-tune models to prevent model drift are either ‘very ineffective’ or ‘somewhat ineffective’. Over half (57%) said that a lack of monitoring to measure the impact of models once deployed was a significant barrier to the adoption of responsible AI.
The right steps to reaping the benefits
To reap the benefits of AI in the long term will require the following measures:
-
Integrated, scalable standards
It is necessary to define scalable standards for model development that can be seamlessly integrated into existing business processes. These standards should not only take into account the accuracy and performance of AI models; factors such as interpretability, fairness and robustness should also be considered. By introducing firm model development standards and guidelines, organisations can ensure that their AI initiatives align with evolving industry requirements and integrate seamlessly into the various facets of their business. Moreover, they need to demand that AI developers follow the standards.
-
The application of blockchain technology
The ethical concerns surrounding AI require the establishment of mechanisms to monitor and maintain ethical AI modelling standards. The use of technologies such as blockchain can provide a transparent and immutable record of the decision-making process within AI models. Blockchain can be used to create a decentralised and secure record of the entire lifecycle of an AI model, including its development, training data and subsequent fine-tuning. This not only ensures traceability and accountability; it also prevents unauthorised changes to the model.
Using blockchain technology to record the decision-making process in AI models transparently and immutably can also increase trust in the technology. The accountability of developers and operators is increased, enabling traceability and auditing of AI systems and ensuring that model development standards, governance, and regulation are always met.
-
Comprehensible machine learning architectures
It is also essential to invest in interpretable machine learning architectures. These prioritise transparency and explainability in AI models, making it possible to understand the algorithms’ decision-making processes. This promotes trust among users and customers while facilitating regulatory compliance and ethical review. Focusing on interpretable architectures for machine learning is a proactive step towards building a sustainable and responsible AI ecosystem where stakeholders can utilise the capabilities of the technology in a trustworthy and ethical manner.
Building a culture of ethical AI
There is no doubt that the effective use of responsible AI will help optimise customer experiences and outcomes at every step of their business interactions. The list of real-time applications of AI in practice continues to grow and while financial organisations are already using AI creatively and efficiently, responsible AI practices that ensure trust and compliance with regulations will extend the benefits further.
Transparency, fairness, data protection, regulatory compliance, ethical decision-making frameworks and a culture of ethical AI must be integral parts of a corporate strategy when using artificial intelligence. By overcoming such challenges, financial institutions can reap the benefits of AI innovation, as well as contribute to building a more responsible and trustworthy financial ecosystem.
For more information, see my recent presentation at FICO World 2024, where I discussed these issues in a short talk.
Dr. Scott Zoldi is chief analytics officer at FICO.
He is responsible for artificial intelligence (AI) and analytic innovation across FICO’s product and technology solutions. While at FICO he has authored more than 130 analytic patents, with 96 granted and 40 pending. Scott is an industry leader at the forefront of Responsible AI, and an outspoken proponent of AI governance and regulation.