It has been over a year since ChatGPT was launched, and more people than ever have been talking about how Large Language Models (LLMs) could change industries worldwide. Regulatory technology (RegTech), primarily concerned with enhancing regulatory processes through technology, could find a promising ally in Machine Learning (ML) and, in particular, LLMs. It is essential to approach this frontier with a balanced perspective, recognising the significant challenges ahead.
Some of the potentials
One of the most significant impacts of LLMs in payment regulatory/compliance technology or RegTech is the potential for enhanced compliance and risk management. LLMs can be helpful in areas such as monitoring transactions for anti-money laundering (AML), performing risk assessment or monitoring adherence to compliance.
LLM’s ability to process vast amounts of textual unstructured data makes them ideal for identifying suspicious patterns or anomalies that may indicate money laundering activities. They also can be used to create realistic training simulations for AML professionals, helping them to recognise and respond to complex money laundering schemes.
In the risk domain, LLMs can assist in assessing the risk levels of clients by analysing vast amounts of unstructured data from various sources, including public records, news articles, and social media, to identify potential risks that traditional structured data analysis might miss.
Another area in which LLMs can be used is to automate and enhance the efficiency of compliance checks. This can be accomplished by analysing the content of communications, such as emails and messages, against regulatory requirements and internal policies to detect non-compliance issues more effectively.
Addressing concerns
Alongside these opportunities for positive benefits, there are also challenges and concerns. The first is LLMs’ dependence on training data quantity and quality. The efficacy of LLMs is heavily dependent on the quality of the input data. LLMs are trained on large datasets of text and code, which may contain biases – if these biases are not addressed, LLMs could perpetuate them in their outputs. In addition, financial crimes and regulatory environments are constantly evolving. LLMs trained on historical data might not remain relevant or accurate over time, leading to model drift. Therefore, continuous monitoring and updating of models are necessary to maintain their effectiveness.
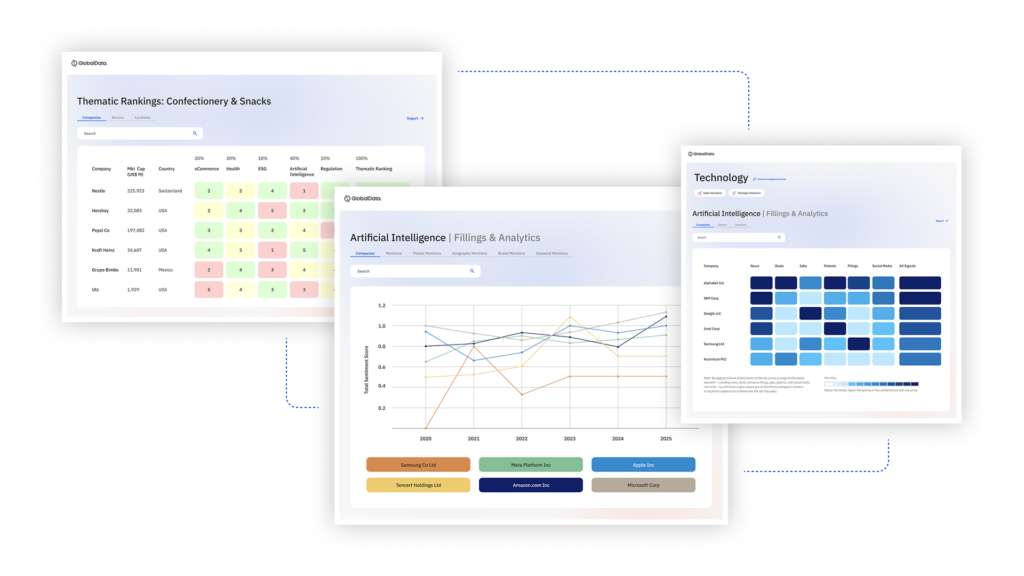
US Tariffs are shifting - will you react or anticipate?
Don’t let policy changes catch you off guard. Stay proactive with real-time data and expert analysis.
By GlobalDataAnother challenge is explainability. It is difficult to explain how LLMs make decisions, making it hard to know how to hold them accountable for their outputs. These models process and generate human-like text by analysing and mimicking patterns found in massive datasets of language. However, the internal workings of LLMs are complex and often opaque, making it difficult to trace how specific outputs are derived from given inputs. This is especially challenging when LLMs produce nuanced text interpretations or when they generate new content, as the reasoning behind these processes can be intricate and multi-layered.
Finally, there could also be a looming risk of over-reliance and over-trust in LLMs, leading to a possible skills gap in the workforce. As machines are increasingly capable of taking over routine tasks, the need for human oversight and understanding of the regulatory landscape will remain vital. This shift towards automated systems raises concerns about workforce skills becoming obsolete, highlighting the importance of continuous learning to ensure that human expertise keeps pace with technological advancements.
Looking ahead
It is also essential to consider several other aspects, such as the maturity of LLM technology. Being relatively new, there’s a risk that it may not yet be sufficiently developed for widespread adoption in RegTech contexts. Also, the impact of LLMs will likely differ depending on the specific regulatory environment in which they are deployed.
Another critical factor is the size and resources of a firm. Larger firms with more resources are generally better positioned to adopt and benefit from LLMs than smaller firms. Firms with experienced legal and compliance teams are more likely to understand and effectively mitigate the risks associated with using LLMs. This disparity underscores the need for scalable solutions that can help smaller firms enjoy the benefits of LLMs, ensuring a more level playing field in the evolving landscape.
Also, as LLMs take on more tasks, the human workforce needs to focus on areas where human judgement and oversight are irreplaceable. A hybrid model that combines the strengths of LLMs with human expertise could be the way forward – to leverage the efficiency and analytical power of LLMs while retaining humans’ critical thinking and ethical judgement.
In conclusion, while the journey of integrating LLMs into RegTech is filled with both promise and complexity, responsibly moving in this path can lead us to a future where efficiency and accessibility are enhanced. The key will be to blend the power of technological innovation with the invaluable insights and judgement that only human experience can provide.
Fatemeh Nikayin is co-founder of Rivero