Banks are operating in an unprecedented environment,
with the amount of data increasing exponentially. Research from
Oracle examines traditional approaches to data warehousing, how
many of them failed during the financial crisis, and discusses the
resulting requirements and marketplace demands now
prevalent.
Among many lessons learned as a result of the economic crisis,
it is becoming crystal clear that critical decision-making hinges
on the quality of analytical data in financial services.
This is, in turn, completely
dependent on the robustness of the data management processes and
infrastructure within the bank.
Banks also no longer have the
luxury of large multi-year initiatives to identify and address data
management issues using a ‘big-bang/big data project’ approach.
There is an increasing need for
them to respond quickly, and accurately with the right supporting
analytical data, be it to regulators or their own management.
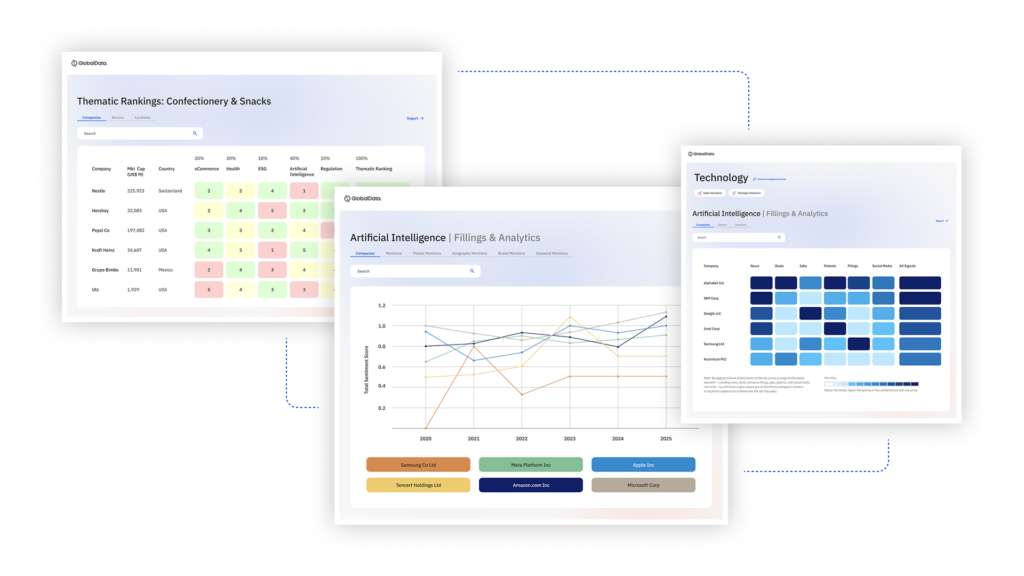
US Tariffs are shifting - will you react or anticipate?
Don’t let policy changes catch you off guard. Stay proactive with real-time data and expert analysis.
By GlobalDataS Ramakrishnan, group
vice-president and general manager, Oracle Financial Services
Analytical Applications, tells RBI industry issues such as
regulatory changes, stress testing, capital adequacy risk
adjustment performance are not new problems in themselves, but have
to be solved in a new way.
“‘What if?’ computations have to be
found within very little turnaround time. The landscape definitely
has changed.”
While there is ongoing debate about
the ultimate causes of the crisis, and the set of possible
remedies, one of the key lessons is the role played by information
systems, particularly analytical systems.
The consequences are far reaching.
Banks are now faced with the prospect of increasing, detailed
regulatory scrutiny, such as that found in Dodd Frank and Basel
III, to complement the existing regulatory regimes already in
place.
This results in even greater stress
on their operations and analytical systems to adapt and respond
accordingly.
Given the lack of preparedness
highlighted in the crisis, financial services institutions are
realising that a fundamental rethink of data management approaches
and practices is necessary.
Another set of equally important
drivers facing banks is the nature of the competitive landscape,
and how technology advances have altered it.
For example, there is increasing
pressure for banks to support their operations with analytical
information that can be delivered in time for in-transaction
decision-making.
This is in stark technological
contrast to traditional, ‘offline’ analytical applications used
solely to support senior management decisions.
Additionally, real-time pricing,
real-time decisions in CRM, fraud detection and surveillance and
algorithmic trading all represent an emerging class of analytical
workflows that are needed ‘on-demand’ and where the deliver of
so-called ‘business intelligence’ is no longer a periodic activity
within a defined time window.
Data warehouses have always been
considered as part of the solution to the data management problem,
not just in financial services.
Ever since the discipline was
founded, it has come to represent a popular set of solution
patterns applicable to data management for analytical end uses.
So, what is the problem?
According to Oracle, the
fundamental reason for the high failure rates for data warehousing
in financial services can be attributed to a lack of understanding
of the end uses of a data warehouse within the institution.
Analytical needs in financial
services are arguably the most complex, varied and fastest evolving
compared to other industries.
The sheer number of existing
regulatory, competitive and operational drivers as well as the
computational complexity of analytical techniques and the
associated data flows, has resulted in a proliferation of
analytical silos where specialised solutions are deployed.
Only sourcing data is relevant to
them and is often with little or no regard for a coherent
overarching data management strategy.
Against this backdrop,
‘traditional’ data warehousing places emphasis on generic patterns
and approaches to provide a data foundation for analytics without
particular reference to the problems unique to financial
services.
This mismatch is the key
contributor to the questionable success in applying these
techniques to financial services institutions.
According to Oracle, there is a
necessity to rethink standard data warehouse practices as they are
applied in banking industry.
The core underlying principle
driving the data warehouse should not be ‘data for data’s sake’ but
a clear, unwavering focus on the end uses supported by the data
warehouse environment.
But the key overarching theme, says
Ramakrishnan, is that the discipline of data warehousing in
financial services is shifting fundamentally – from an assembly of
generic components and tools towards a specialised platform
approach that supports the unique analytical needs of financial
services institutions worldwide.
Rather than merely being a provider
of operational/business data for downstream analytical consumers,
the warehouse should be a single foundation that supports
end-to-end analytical processing including data sourcing,
calculation and aggregation processes and results/reporting for
every use case.
Custom assembly of a
data-warehousing environment has historically proven costly and
prone to high failure rates partially because of ill-defined and
often overreaching scope.
A more reasonable way to address
this problem is to utilise a unified analytical platform that can
support all the key requirements and usage patterns of a typical
financial services institution – rather than attempt to combine
general-purpose tools to achieve when facing next generation data
warehousing projects.
In conclusion, Ramakrishnan argues
that putting the right data warehousing strategy in place can
provide IT organisations in the industry with a unified analytical
platform that will enable them to support both current and future
analytical requirements in a cost-effective, scalable and flexible
manner.
“The regulators are coming calling
and banks have to do something. There is a mountain to climb,” he
says.
“Banks have to think about what
they want to do with data and think about it now and make sure it
runs inside a responsive analytical architecture or
infrastructure.
“But on a positive note, even for a bank with, say 30m retail
banking customers, it is now possible to solve classes of data
problems that until recently they would think was outside their
reach.”